Computer vision and labelling tool for interpreting image data
EarthNET AI Images enables you to interpret image data faster, cheaper and in a more data-driven manner with uncertainty quantified at each step. With generic applicability across images of different types, it includes functionality for image classification, object detection, unsupervised image segmentation, semantic embedding, semi-supervised segmentation and supervised segmentation.
- OBJECT DETECTION
- IMAGE CLASSIFICATION
- IMAGE SEGMENTATION
- MODEL-ASSISTED LABELING
- PRE-TRAINED MODELS
- UNCERTAINTY MEASURES
Object detection
Use the object detection functionality in EarthNET AI Images to identify the class and location of objects within images. This method is well-known from applications such as facial recognition and identifying objects in street images.
In geoscience, this computer vision method can be used to classify, locate, and count different types of rock. You can simply outline classified objects with rectangular bounding boxes. This can also be expanded to instance segmentation, where objects are precisely outlined with segmentation masks.
Image classification
Use the image classification functionality in EarthNET AI Images to assign a class to each image. During image classification of rock samples, the labelling and deep-learning prediction steps can be supported by the combination of image data with a rich suite of metadata including, stratigraphic information, elemental composition (XRF), mineralogical composition (XRD) and more.
Image segmentation
Use the image classification functionality in EarthNET AI Images to to extract very detailed information from images. Our detailed workflow for image segmentation includes rapid annotation, unsupervised segmentation, semantic embedding, and training of supervised segmentation models.
Compute percentages of minerals and lithologies from the segmentation mask and transfer the data to the well-log domain, enabling integration of image data with the well-log and seismic data.
Model-assisted labeling
Labeling data can be a time-consuming and expensive task in geoscience work. EarthNET AI Images provides model-assisted labeling functionality that speeds up the labeling process by using pre-trained machine learning models. Our models perform the initial labeling, and human experts review the results and make adjustments if needed.
Another means to speed up data annotation in EarthNET is through programmatic labeling, where you assign appropriate classes using rules based on existing labels, model predictions, and metadata.
Pre-trained models
EarthNET AI Images provides you with pre-trained models for cuttings and core images. These models provide a reliable and efficient interpretation of image data with just one click. You can also build upon the pre-trained models to improve their capabilities, instead of building a model from scratch. Our pre-trained models include lithology classification, lithology segmentation, grain size classification, and sand type classification.
Uncertainty measures
The multiple interpretation modes in EarthNET AI Images enable you to quantify the uncertainty in their data. We provide a confusion matrix that allows for comparison of input and output from a model, or comparison of outputs from two models. Easily filter by input/output disagreement to identify errors and problems that need to be corrected through additional labeling.
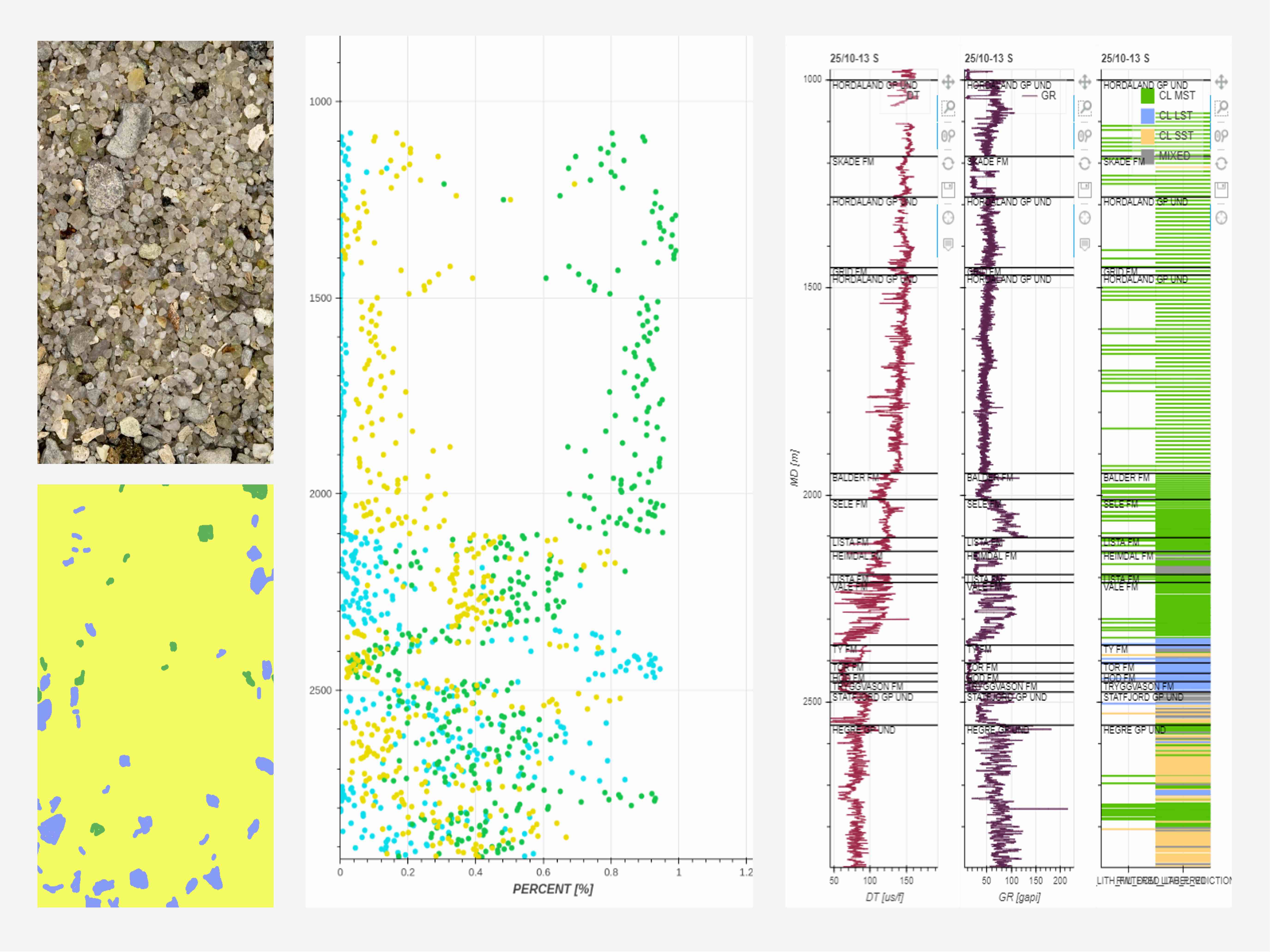
Improve geoscience workflows by incorporating rock samples
Interpreting rock samples is a crucial aspect of geoscience as they provide the closest representation of the ground truth. Usually this process is done manually under suboptimal conditions, leading to errors and inefficiencies. Since the interpretation is time-consuming and expensive, it is often limited to few samples despite vast amounts of data available.
With EarthNET AI Images, you can interpret rock samples with speed and accuracy. Incorporating these interpreted rock samples into your workflows increases your understanding of reservoir formations and improves well planning, petrophysics, and sedimentology studies.
Unlock the dormant value of your image data
EarthNET AI Images offers a comprehensive solution for managing and analysing image data. Automatic metadata tagging and advanced computer vision techniques enables you to easily label and train ML models on your data, and through our active learning methods you can continuously improve both the label sets, models, and predictions.
Ingest your image data and automatically pair it with a large number of metadata tags.
You can label your images by using different computer vision techniques or by using pre-trained models.
Train an AI model on your labeled data. Use cloud computing for efficient model training.
Apply the model to all images. View the results in the metadata table and visualise it in EarthNET Viewer.
Evaluate your model results and detect potential errors by filtering model predictions together with available metadata.
Use active learning methods to iteratively improve the label set, model and predictions.
"The cuttings interpretation is very accurate and allows us to map out proximal-distal trends in our reservoir formation, bringing new value from a previously underutilised data set."
Explore our resources
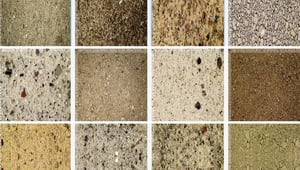
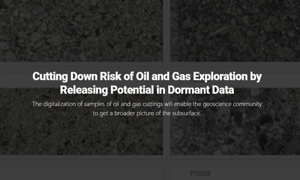
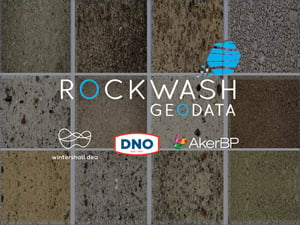