AI-powered data analytics tool for interpreting well data
EarthNET AI Wells uses Machine Learning models to predict reservoir properties directly from well logs, core data, fluid data and CPI’s with speed and precision.
- WELL DATA ANALYSIS
- DATA CLEANING
- DATA LABELING
- MODEL TRAINING
- PRE-TRAINED MODELS
- MODEL QC
- ACTIVE LEARNING
Well data analysis
EarthNET AI Wells offers a suite of powerful tools to help you visualise and explore your well data.
With these tools, you can easily identify issues like biases, skewed distributions, gaps, and unit problems in your data that need to be resolved before starting the machine learning interpretation workflow.
Data cleaning
EarthNET AI Wells streamlines the data preparation process and ensures that your models are trained on high-quality data.
Our data cleaning capabilities lets you remove non-physical values, ensure consistent units, and fill in missing information in your well data. In EarthNET AI Wells includes functionality for flagging and clipping bad log values, de-spiking logs, removing linear interpolation and manually editing logs.
Data labeling
EarthNET AI Wells provides both unsupervised and supervised machine learning capabilities. For supervised learning, the models learn from labels that represent the ground truth.
You can either import labels from available sources such as CPI logs, or you can create them using the labeling tools available in EarthNET.
Model training
Train ML models from scratch to predict your property of interest, including DTS, lithology, porosity, water saturation and more.
In EarthNET AI Wells you get access to a wide range of state-of-the-art ML model architectures, including neural networks such as MLPs, Unet’s and transformers, and tree-based methods such as random forests and XGBoost.
Pre-trained models
Speed up your interpretation by more than 10x and trigger predictions with a single click using our pre-trained AI models. EarthNET AI Wells provides you with pre-trained models for predicting shear sonic, lithology, porosity and water saturation.
Model QC
Review and compare model results and performance metrics for multiple models to identify the models that perform best for your tasks.
All your trained ML models will be stored in your model library, along with all the metadata that describes the training data used, the model architecture, parameters, and results. With full information about each model, you can quickly identify potential causes for suboptimal performance, mitigate them and re-train the model.
Active learning
The infrastructure in EarthNET facilitates active learning to ensure that you have a set of models that improve over time with minimal effort.
EarthNET supports active learning workflows through quantifying model performance, improving input data through data cleaning and labeling, retraining models using the improved input data, and tracking model performance over time.
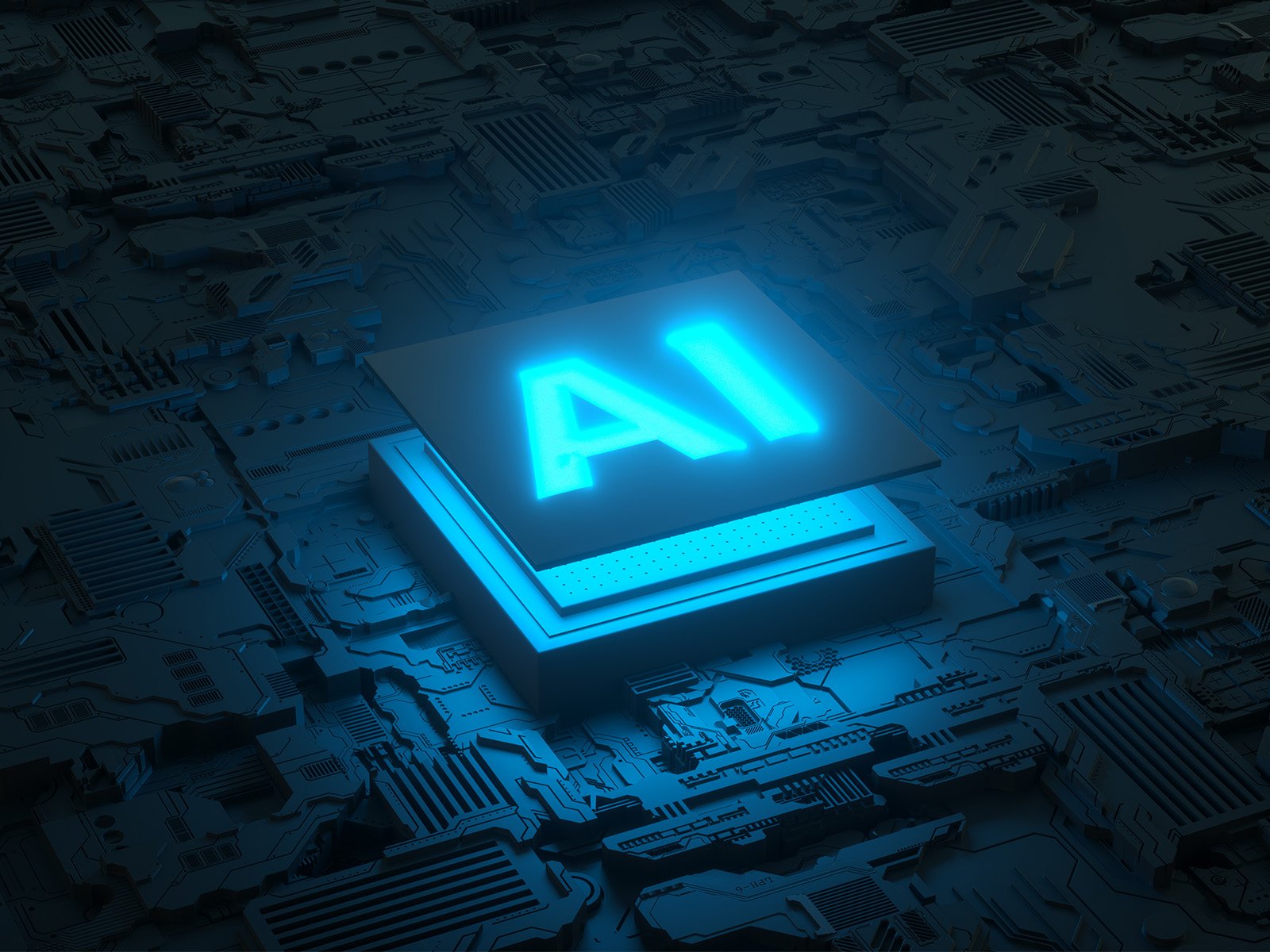
From prospect-level accuracy to basin-level coverage
Traditional well logs measure physical properties, but we're interested in the lithology, porosity, permeability, and water saturation of rocks. Accurately quantifying these properties is crucial to understanding hydrocarbon accumulations and CO2 storage capacity. It is, however, very time-consuming, and it can take a petrophysicist 1-2 days to interpret one single well.
EarthNET AI Wells revolutionises your petrophysics by enabling you to predict rock- and fluid properties with AI - faster, better, and cheaper than traditional methods, with uncertainty quantified at each step. Doing a large-scale study of 5000 wells would take 13-27 years using traditional methods compared to 3 months using EarthNET AI Wells.
Accelerating your well interpretation workflows
EarthNET AI Wells provides a comprehensive overview of your well data, allowing you to easily identify errors, make necessary corrections, and perform advanced data analytics using cutting-edge AI models.
Get full overview of your well data to identify errors or inconsistencies
Remove non-physical values and fill in missing logs
Select existing labels or create labels using our labeling tools
Train a model from labels or build upon a pre-trained model
QC model metrics and review predictions against labels
Use the model to make predictions on the complete data set