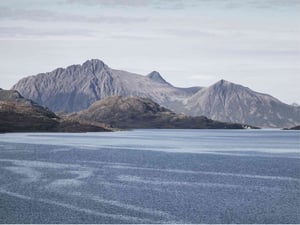
CASE STUDY
Characterising and de-risking
a carbon storage site using AI
The process of identifying, characterising, and de-risking suitable sites for CO2 storage requires robust and reliable 3D mapping of subsurface reservoir properties to ensure that decisions to develop such sites are based on reliable information. The urgency of mitigating global warming via reduced emissions of CO2 to the atmosphere requires that we develop more efficient and cost effective workflows. Earth Science Analytics is working with partners such as Horisont Energi to achieve both these requirements by leveraging geoscience data and AI methods.
Characterising and de-risking of a carbon storage site in 3D
The Norwegian Barents Sea
Rapidly and accurately assess rock and fluid properties for a carbon storage site
The success of carbon capture and storage (CCS) relies heavily on the ability to rapidly identify suitable carbon storage sites and accurately assess their properties. Due to the sparse amount of data available over suggested storage sites, it is challenging to accurately assess the physical rock and fluid properties of candidate reservoirs, which significantly impact the safe operation of carbon storage sites.
AI-assisted geoscience software for CCS site characterisation and de-risking
Our AI-driven software, EarthNET, enables characterisation and de-risking of carbon storage sites with speed and precision. By using EarthNET, we can predict properties from wireline logs to increase data coverage, use available nearby well and seismic data to increase prediction confidence in the area of interest, and integrate this data to predict rock properties in 3D.
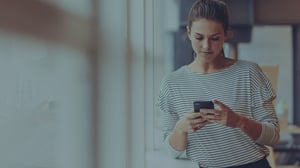
"By utilising ESA's deep domain expertise in both geoscience and deep learning technology, we were able to augment a sparse dataset over the area of interest with additional relevant geological data. With its EarthNET technology, ESA captured regional trends in geology, quantified the full range of uncertainty within acoustic and physical properties, and provided a data-driven model that challenges underlying assumptions within traditional workflows."
Full site characterisation and quantification of uncertainty within acoustic and physical properties
EarthNET enabled predicting properties from wireline logs to increase data coverage, using available nearby well and seismic data to increase prediction confidence in the area of interest, and integrating this data to predict rock properties in 3D.
ESA’s machine learning capabilities reduce interpretation cycle time and allow more time to be spent on risk assessment and management. Using the EarthNET technology saves time, improves results, and enables working on a previously unfeasible scale. As a result, the operator and partners can now spend more time on fully understanding the potential challenges this storage site may pose.
EarthNET enables working at a previously unfeasible pace, allowing more time to be spent assessing and managing the risk this storage site may pose.
Interpretation cycle time was drastically reduced from years to months through the use of the AI-assisted interpretation modules in EarthNET.
By using AI models trained on vast amounts of QC'ed data, human bias and subjectivity is reduced and the accuracy of the interpretation increased.